Summary: A new AI model that mimics the neural architecture and connections of the hippocampus is able to alter its synaptic connections as it moves a car-like virtual robot.
Source: Human Brain Project
HBP researchers at the Institute of Biophysics of the National Research Council (IBF-CNR) in Palermo, Italy, have mimicked the neuronal architecture and connections of the brain’s hippocampus to develop a robotic platform capable of learning as humans do while the robot navigates around a space.
The simulated hippocampus is able to alter its own synaptic connections as it moves a car-like virtual robot. Crucially, this means it needs to navigate to a specific destination only once before it is able to remember the path.
This is a marked improvement over current autonomous navigation methods that rely on deep learning, and which have to calculate thousands of possible paths instead.
“There are other navigation systems that simulate the role of the hippocampus, which acts as a working memory for the brain. However, this is the first time we are able to mimic not just the role but also the architecture of the hippocampus, down to the individual neurons and their connections,” explain Michele Migliore and Simone Coppolino of the IBF-CNR, who published their findings in the journal Neural Networks.
“We built it using its fundamental building blocks and features known in literature – such as neurons which encode for objects, specific connections and synaptic plasticity.” Taking inspiration from biology, the researchers were able to use different sets of rules for navigation than the ones used by deep-learning-driven platforms.
To reach a specified destination, a deep-learning system calculates possible paths on a map and assigns them costs, eventually choosing the least expensive path to follow. It is effectively based on trial and error and require extensive calculation: decades of studies have been dedicated to reducing the amount of work for the system.
“Our system, on the contrary, bases its calculation on what it can actively see through its camera,” the researchers explain.
“When navigating a T-shaped corridor, it checks for the relative position of key landmarks (in this case, coloured cubes). It moves randomly at first, but once it is able to reach its destination, it reconstructs a map rearranging the neurons into its simulated hippocampus and assigning them to the landmarks. It only needs to go through training once to be able to remember how to get to the destination.”
This is more similar to how humans and animals move – when you visit a museum, you first wander around the place, not knowing your way, but if you then need to go back to a specific exhibit, you immediately remember all the steps needed.
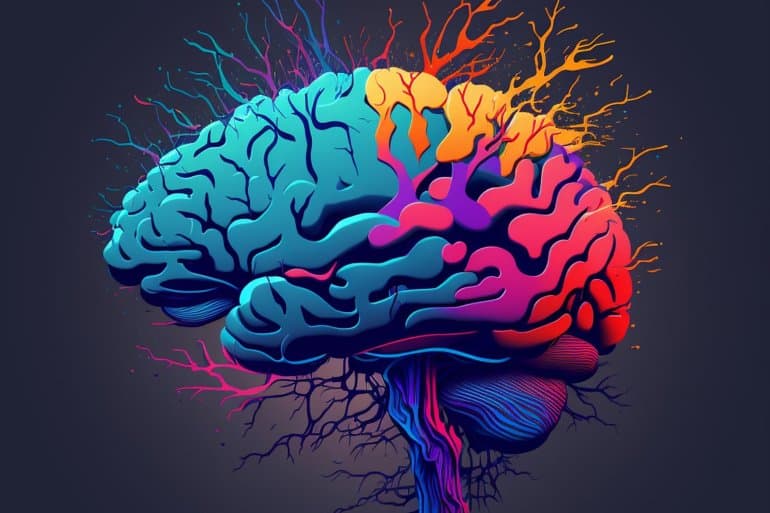
Both the robotic platform and the hippocampal simulation have first been implemented through the digital research infrastructure EBRAINS, which then allowed the researchers to build and test a physical robot in a real corridor.
“Object recognition was based on visual input through the robot’s camera, but it could in theory be calibrated on sound, smell or movement: the important part is the biologically inspired set of rules for navigation, which can be easily adapted to multiple environments and inputs.”
Another member of Migliore’s lab, Giuseppe Giacopelli, is currently working on making the system tailored for industrial use by encoding for the recognition of specific shapes.
“A robot working in a warehouse could calibrate itself and be able to remember the position of shelves in just a few hours,” says Migliore. “Another possibility is helping the visually impaired, memorizing a domestic environment and acting as a robotic guide dog.”
About this artificial intelligence research news
Author: Roberto Inchingolo
Source: Human Brain Project
Contact: Roberto Inchingolo – Human Brain Project
Image: The image is in the public domain
No hay comentarios:
Publicar un comentario