- 1New York Genome Center, New York, NY, United States
- 2Deparatment of Physiology and Biophysics, Institute for Computational Biomedicine, Weill Cornell Medicine, New York, NY, United States
El reciente auge de la genómica unicelular, que incluye el desarrollo de una amplia gama de enfoques experimentales y computacionales, ha permitido comprender las complejas redes moleculares de las células durante el desarrollo y en las enfermedades humanas con una resolución sin precedentes. El análisis del transcriptoma unicelular ha permitido la investigación de alta resolución de la heterogeneidad celular en una amplia gama de poblaciones celulares, desde embriones tempranos hasta tejidos complejos, con el riesgo de captar sólo una imagen parcial de las complejas redes moleculares de las células. Las tecnologías multiómicas unicelulares pretenden colmar esta laguna proporcionando una visión más holística de la célula mediante la medición simultánea de múltiples tipos moleculares de la misma célula y ofreciendo una visión más completa de las interacciones y funciones combinadas de múltiples capas reguladoras a resolución de tipo celular. En esta revisión, resumimos brevemente los avances recientes en las tecnologías unicelulares multimodales y analizamos los retos y oportunidades de este campo.
Introduction
El genoma es el modelo de todas las células vivas y contiene la información necesaria para la producción de ARN y proteínas, las moléculas funcionales responsables de los diversos procesos biológicos de las células. Los análisis genómicos, que incluyen el perfil de las variaciones en las secuencias del genoma, los estados epigenéticos de los elementos reguladores y la abundancia de ARN, han permitido comprender mejor los principios fundamentales que rigen los procesos moleculares de la célula. Sin embargo, el análisis de una mezcla de poblaciones celulares da lugar a señales promediadas de diversos tipos celulares, lo que oscurece las variaciones entre células de los estados moleculares, que son cruciales para las funciones biológicas adecuadas en los organismos pluricelulares. Los recientes avances en genómica unicelular permitieron la disección de programas moleculares específicos de cada tipo celular a partir de entornos celulares complejos mediante el aislamiento y análisis de células individuales (Papalexi y Satija, 2018). Por ejemplo, los programas transcripcionales dinámicos están estrechamente vinculados a la identidad y los estados funcionales de las células; analizar el transcriptoma a nivel unicelular permite interrogar los programas de expresión compartidos y distintos de las células (Tang et al., 2009). La metilación del ADN es una marca epigenética que regula y mantiene los programas transcripcionales específicos de cada tipo celular. El análisis del metiloma del ADN de células individuales permitió evaluar la heterogeneidad epigenética (Smallwood et al., 2014)
Los elementos reguladores específicos de cada tipo celular están asociados a la accesibilidad de la cromatina, y diferentes combinaciones de modificaciones de las histonas conducen a la activación o represión de los genes; los módulos de elementos reguladores coordinados podrían identificarse mediante la medición unicelular de los estados de la cromatina (Buenrostro et al., 2015; Cusanovich et al., 2015; Rotem et al., 2015). Las estructuras cromosómicas de alto orden se han relacionado con la regulación génica y la replicación y reparación del ADN. La asociación de los programas transcripcionales del territorio cromosómico.
El análisis del metiloma del ADN de células células individuales permitió evaluar la heterogeneidad epigenética (Smallwood et al., 2014). Las variabilidades de la estructura con los patrones de activación del genoma se revelaron mediante la captura de la estructura cromosómica en células individuales (Nagano et al., 2013).
However, measuring one modality at a time only captures the partial picture of the complex molecular network, while the intricate interactions and combined effects from multiple molecular layers could be lost. Therefore, measuring multiple molecular layers jointly from the same cells is crucial for gaining a more complete understanding of these complex molecular programs of the cells. Building on stand-alone single-cell genomics technologies, single-cell multimodal omics approaches have recently been developed to perform multiple measurements from the same cells (Figure 1). Computational approaches were also developed to perform multimodal integration of associated measurements to identify the relationships and crosstalk between multiple variables, enhancing our understanding of the determination and maintenance of cellular states (Chappell et al., 2018; Efremova and Teichmann, 2020; Zhu et al., 2020).
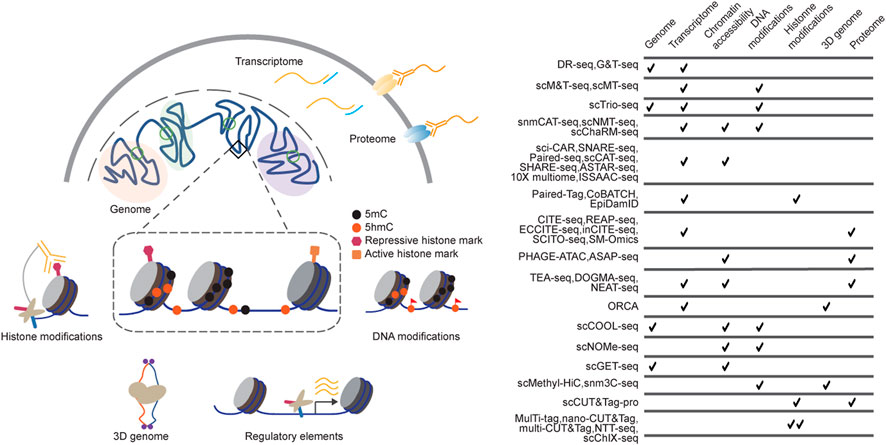
FIGURE 1. Technologies for single-cell multimodal measurements. (A) Single-cell multi-omics methods have been developed to profile DNA sequences, chromatin states, and gene expression from the same cells. Single-cell multimodal omics methods are designed on various strategies, including Tn5 tagmentation, proximity ligation, immunoprecipitation, and chemical labelling, among which they perform high biocompatibility and orthogonality; (B) the table summarizes some of the current single-cell multi-omics sequencing methods.
Linking genome with transcriptome
Genomic variation is the differences in DNA sequence among individuals or populations and could contribute to abnormities, such as anosmia and complex neurological diseases (Hasin-Brumshtein et al., 2009; Zhang et al., 2009). Joint analysis of genome and transcriptome enables a better understanding of how genetic variations affect the behaviors of individual cells and therefore holds great promise for understanding the biology of cancers (Group et al., 2020). Build on single-cell WGA (whole genome amplification) (Zong et al., 2012) and RNA sequencing methods (Hashimshony et al., 2012), DR-seq (gDNA-mRNA sequencing) (Dey et al., 2015) and G&T-seq (genome and transcriptome sequencing) (Macaulay et al., 2015) have been developed to profile the genome variation and transcriptome simultaneously. The relationships of chromosomal aneuploidies and interchromosomal fusions with the variabilities of gene expression among individual cells were revealed by these analyses at single-cell resolution. Additionally, these methods could be used for the assessment of the origin and evolution of tumors by lineage tracing with enhanced sensitivity via tracking both genomic DNA and massager RNA at the single-cell level (Wagner and Klein, 2020).
Chromatin states and gene expression
Chemical modifications on DNA bases, such as cytosine methylation (5 mC), can alter the activity states of cis-regulatory elements (CREs) by facilitating or inhibiting the binding of transcription factors to CREs in a cell type-specific manner (Smith and Meissner, 2013). DNA modifications are associated with various biological processes, including development, disease, and aging (Greenberg and Bourc’his, 2019). Based on the optimized bisulfite treatment to achieve base-resolution detection of 5 mC from a limited amount of input materials, methods for single-cell parallel profiling of the methylome and transcriptome have been developed to dissect the relationships between gene expression and 5 mC modification levels in functional genomic regions (Angermueller et al., 2016; Hou et al., 2016; Hu et al., 2016; Luo et al., 2022). ScTrio-seq further links genomic copy-number variations with DNA methylome and transcriptome in individual cells and dissects the regulatory heterogeneity in hepatocellular carcinomas (Hou et al., 2016). However, these methods depend on the physical separation of cytoplasm RNA and nucleic genome DNA (gDNA) and run into the risk of material loss. The recently developed snmCAT-seq incorporates 5-methyl-dCTP during reverse transcription of RNA—the resulting cDNA are resistant to bisulfite treatment, and thus, the “C-to-T” signal could be used to identify genome DNA fragments from cDNA derived from mRNA without the need of separating mRNA and gDNA prior to amplification (Luo et al., 2022). Their integrative approach enabled the reconstruction of the regulatory hierarchy for 63 human cortical cell types and the prediction of cell types that are associated with diseases.
To identify the location of CREs, assays including DNase-seq (Boyle et al., 2008), MNase-seq (Schlesinger et al., 2013), and ATAC-seq (Buenrostro et al., 2013) were developed to profile chromatin accessibilities. ATAC-seq uses the hyperactive Tn5 transposase to perform simultaneous fragmentation and tagging of open chromatin regions for subsequent library amplification and sequencing. Based on this streamlined sample-to-sequencing library workflow, single-cell ATAC-seq has been developed and widely used to map candidate cis-regulatory elements to resolve cell type-specific usage of “regulatome” (Cusanovich et al., 2015; Mezger et al., 2018; Lareau et al., 2019). Combining scATAC-seq with various single-cell indexing platforms, including combinatorial barcoding and microfluidics systems, high-throughput methods for joint analysis of chromosome accessibility and transcription were developed to reveal the associations between regulatory elements and putative targeted genes. These methods demonstrated their utilities in gaining a global view of the cellular composition and cell type-specific molecular programs in healthy tissues and diseases by the parallel generation of epigenome and transcriptome maps from a large number of single cells (Cao et al., 2018; Lake et al., 2018; Chen et al., 2019; Liu et al., 2019; Zhu et al., 2019; Ma et al., 2020; Xu et al., 2022). For example, sci-CAR and Paired-seq linked distal CREs to potential target genes with the covariance of chromatin accessibility and transcription across single cells (Cao et al., 2018; Zhu et al., 2019); SHARE-seq measures the chromatin potential of single cells and enables the finer dissection of closely related cellular states compared to scRNA-seq (Ma et al., 2020).
The active or repressive roles of regulatory elements are defined by their epigenetic states, such as different combinations of histone modifications and binding of transcriptional factors. Chromatin immunoprecipitation followed by sequencing (ChIP-seq) is the gold standard for analyzing chromatin-protein interactions, while the requirement for a large amount of input materials makes single-cell ChIP-seq analysis challenging (Rotem et al., 2015). CUT&Tag based on in situ tagmentation guided by antibodies targeting histone modifications or transcription factors of interest significantly reduced the input requirements and enabled the investigation of histone modifications at the single-cell level (Kaya-Okur et al., 2019; Bartosovic et al., 2021; Wu et al., 2021). However, different histone mark datasets may have varying resolutions in separating cell groups - due to both technical factors (such as antibody efficiencies and specificities) and their distinct biological functions (such as their genome distribution patterns and relationships with gene expressions), and thus computational integration of multiple different histone modification datasets is challenging. Jointly profiling of histone modifications with gene expression could allow unbiased integration of different chromatin states datasets using transcriptome as the central modality (Xiong et al., 2021; Zhu et al., 2021; Rang et al., 2022). Such strategies could also be applied to predict the putative target genes for both the active and repressive regulatory elements at cell-type resolution in complex tissues (Zhu et al., 2021).
Towards higher-order chromosome organization
In addition to the epigenetics states of regulatory elements, the three-dimensional organization of the genome also makes key contributions to the regulation of gene expressions. Chromosome conformation capture sequencing methods were developed to map the interactions between distal chromatin regions and provided insights into how the genome is organized and how they regulate gene expression programs (Lieberman-Aiden et al., 2009). Single-cell Hi-C techniques capture the snapshots of 3D genome organization 1 cell at a time and reveal the dynamic and distinct chromosome conformations during different cell cycle phases and cell types (Nagano et al., 2013; Ramani et al., 2017; Stevens et al., 2017). However, the chromosome conformation profiles do not directly reflect the transcriptional or translational states of cells, and thus identifying and annotating the cell identities from the single-cell Hi-C datasets alone is challenging. To overcome this barrier, methods for co-capturing chromosome conformations with other modalities, such as transcription and DNA methylation in single cells, were developed (Lee et al., 2019; Li et al., 2019; Mateo et al., 2019). Combining RNA-fluorescence in situ hybridization (RNA-FISH) with parallel visualizing of DNA folding in single-cells, ORCA (optical reconstruction of chromatin architecture) revealed physical borders between active and repressed DNA exist in a cell type-specific manner in Drosophila cells (Mateo et al., 2019). Joint analysis of DNA methylation and chromosome conformation revealed coordinated DNA methylation states between distal genomic regions that are in spatial proximity and allowed reconstruction of cell type-specific chromatin organization of complex tissues (Lee et al., 2019; Li et al., 2019).
From RNA to proteins
The transcriptome of cells could function as a central modality for integrating multiple different molecular layers in single-cell multiomics analyses. However, transcriptome analysis only provides information on genes that are being transcribed, but not the abundance of the cell’s functional molecule proteins, and analyzing the functional states of individual cells requires measuring the protein abundances directly. Recently, cellular barcoding of epitopes and transcriptomes emerged as a promising technique for analyzing cell surface proteins of single cells together with transcriptomes (Peterson et al., 2017; Stoeckius et al., 2017). In these methods, cells were incubated with the barcode oligo-conjugated antibodies targeting a panel of cell surface proteins, followed by micro-fluidics capturing both the antibody barcode oligos and mRNA from thousands of single cells in parallel. The cell surface protein abundances can also be jointly measured with histone modifications: by combining CUT&Tag with CITE-seq, scCUT&Tag-pro measures cell surface protein abundances together with histone modifications from the same cell at a time, and scChromHMM integrates different histone modifications use protein abundances as the central modality to generate single-cell “megaomic” profiles, enabling the exploration of heterogeneity in chromatin state across discrete cell types and continuous trajectories (Zhang et al., 2022). These approaches could be further combined with capturing chromatin accessibilities for integrating the epigenome, transcriptome, and protein, providing more complete views on gene regulation (Mimitou et al., 2021; Swanson et al., 2021; Chen A. F. et al., 2022).
Measuring multiple epigenome layers
Another frontier in single-cell multiomics is to dissect the relationships between different regulatory layers and their combined effects on cell function. The combinations of transcription factors and CREs define cell types and developmental trajectories of multicellular organisms. To map multiple chromatin features from the same cells at a time, MulTI-Tag and multi-CUT&Tag pre-barcoded the antibody-tethered transposases targeting multiple chromatin-associated proteins and multiplex them for immunostaining of the cells (Gopalan et al., 2021; Meers et al., 2022). Such analyses resolved unique and coordinated patterns of active and repressive regulatory element usages in distinct cell types and states and allowed analysis of the direct interaction between different chromatin-associated proteins. NTT-seq and nano-CUT&Tag used an alternative strategy of harnessing nanobody-tethered transposases specifically targeting different immunoglobulin-G contain antibodies to pre-barcode antibody-transposase complexes, for the multiplexed chromatin-associated proteins detection (Bartosovic and Castelo-Branco, 2022; Stuart et al., 2022). NTT-seq was also extended for joint profiling of cell surface protein abundances with multifactorial chromatin states, which is particularly useful for understanding the dynamic gene regulation programs in the immune systems (Stuart et al., 2022). By engineering transposase to include the chromodomain of heterochromatin protein-1α, scGET-seq comprehensively assays open and closed chromatin regions and deduced Chromatin Velocity to uncover epigenetic reorganization paths during stem cell reprogramming (Tedesco et al., 2022). The distinct distribution patterns of certain combinations of chromatin-associated proteins could also be utilized to deconvolute their relationships in the same cells. CUT&Tag2for1 obtained the combined signal from H3K27me3 and Pol2S5p and computationally deconvoluted the signals to give high-resolution maps of both the active and repressive regulomes in single cells (Janssens et al., 2022). Benefited from the higher-resolution footprints generated by antibody-tethered MNase, scChIX-seq was able to multiplex and deconvolute additional combinations of chromatin-associated proteins, including H3K27me3/H3K9me3, H3K4me1/H3K27me3, and H3K36me3/H3K9me3 (Yeung et al., 2023).
Multimodal genomics analysis with spatial information
Single-cell multiomics sequencing tools have increased our ability to study the gene regulation mechanisms in tissues; however, the spatial context is lost during tissue dissociation in such analyses. The crosstalk between cells in their native microenvironment is critical for the understanding of the complex cellular networks in health and diseases. To overcome this barrier, a suite of spatial technologies was recently developed for joint analysis of genome, transcriptome, epigenome, and protein abundances. Built on microscopy approaches, optical reconstruction of chromatin architecture (ORCA) linked visualization of DNA folding with RNA in single cells (Mateo et al., 2019) and revealed cell differentiation is associated with extensive 3D remodeling of chromatin structure in developmental control loci. Based on in situ capture of cDNA and antibody tags, SPOTS (Ben-Chetrit et al., 2023) and SM-Omics (Vickovic et al., 2022) performed simultaneous epitope and transcriptome profiling in single cells and enhanced the analysis of differential gene expression programs across tissue regions. By introducing spatial information to single cells with a deterministic barcoding approach, spatial-ATAC-RNA-seq, and spatial-CUT&Tag-RNA-seq enabled co-profiling of accessible chromatin or histone modifications with transcriptome on the same tissue section and uncovered new biological insights in epigenetic priming and gene regulation at different regions of the tissues (Zhang et al., 2023).
Discussions
Single-cell multiomics technologies simultaneously measure multiple types of molecular layers from the same cells, including genome, epigenome, transcriptome, and proteome. These approaches can provide a more comprehensive understanding of the underlying molecular mechanisms governing cellular diversity and function. However, single-cell multiomics is still in its infancy, and several challenges need to be addressed to fully unleash the potential in providing systems understanding of cell’s molecular networks and become more widely adopted by the research community. One key challenge for single-cell multiomics is the balance between data sparsity and throughput. Many current single-cell multiomics approaches are still in the early stages of development and may not be able to capture all the desired molecular layers with high selectivity and sensitivity. The coverages of epigenome and transcriptome for individual cells provided by current high-throughput methods are still low, rendering it difficult to identify cell-to-cell variability from technical noise. Methods for analyzing individual cells could provide much higher sensitivity by saturated measurement, while profiling limited numbers of cells could result in biased representations of the global cellular population. While optimizing existing experimental procedures may help to minimize the gaps, new biochemical methods and approaches may be needed to completely overcome this limitation. The recent drop in sequencing costs has greatly facilitated the generation of single-cell multiomics datasets, and the large-scale analysis and integration of datasets generated by single-cell multiomics technologies have become another major challenge, and future development of computational and bioinformatic methods and tools are also desired (Efremova and Teichmann, 2020). Another area of single-cell multiomics that is less developed is the examination of cellular program changes over time. The use of metabolic labeling to measure newly synthesized mRNA in individual cells was employed to quantitatively analyze the changes in transcription within complex systems (Cao et al., 2020; Qiu et al., 2020). It is likely that this approach could also be adapted to investigate the dynamics of molecular networks during the determination and transition of cell states. An alternative strategy is to simultaneously measure related molecular events, such as the “writing” and “erasing” of epigenetic modifications, to model the forward and reverse rates of cellular reprogramming and to reveal the underlying principles guiding cell state transition, especially during the initiation and progression of human diseases. Finally, future developments for the measurement of multiple molecular layers of single cells without compromising the cell’s viability (Chen W. et al., 2022) could uncover the underlying molecular mechanisms of various cellular processes by linking the initial state of molecular networks with downstream responses.
The field of single-cell multiomics holds great potential and is continually advancing with new experimental and computational approaches to overcome its current limitations. Single-cell multiomics could provide opportunities to identify new therapeutic targets and biomarkers for precision medicine by obtaining comprehensive information on multiple modalities simultaneously. By dissecting the interactions and crosstalk between multiple components within the same cells, single-cell multiomics could also provide insights into the molecular principles for disease establishment and progression and shed light on the future development of treatments.
Author contributions
DB conceived the structure of the manuscript; DB and CZ wrote the manuscript.
Funding
This study was funded by grants from the NIH (4R00HG011483 and 5RM1HG011014 to CZ).
Conflict of interest
The authors declare that the research was conducted in the absence of any commercial or financial relationships that could be construed as a potential conflict of interest.
The handling editor declared a shared affiliation with the author CZ at the time of review.
Publisher’s note
All claims expressed in this article are solely those of the authors and do not necessarily represent those of their affiliated organizations, or those of the publisher, the editors and the reviewers. Any product that may be evaluated in this article, or claim that may be made by its manufacturer, is not guaranteed or endorsed by the publisher.
References
Angermueller, C., Clark, S. J., Lee, H. J., Macaulay, I. C., Teng, M. J., Hu, T. X., et al. (2016). Parallel single-cell sequencing links transcriptional and epigenetic heterogeneity. Nat. Methods 13, 229–232. doi:10.1038/nmeth.3728
Bartosovic, M., and Castelo-Branco, G. (2022). Multimodal chromatin profiling using nanobody-based single-cell CUT&Tag. Nat. Biotechnol. 2022. doi:10.1038/s41587-022-01535-4
Bartosovic, M., Kabbe, M., and Castelo-Branco, G. (2021). Single-cell CUT&Tag profiles histone modifications and transcription factors in complex tissues. Nat. Biotechnol. 39, 825–835. doi:10.1038/s41587-021-00869-9
Ben-Chetrit, N., Niu, X., Swett, A. D., Sotelo, J., Jiao, M. S., Stewart, C. M., et al. (2023). Integration of whole transcriptome spatial profiling with protein markers. Nat. Biotechnol. 2023. doi:10.1038/s41587-022-01536-3
Boyle, A. P., Davis, S., Shulha, H. P., Meltzer, P., Margulies, E. H., Weng, Z., et al. (2008). High-resolution mapping and characterization of open chromatin across the genome. Cell 132, 311–322. doi:10.1016/j.cell.2007.12.014
Buenrostro, J. D., Giresi, P. G., Zaba, L. C., Chang, H. Y., and Greenleaf, W. J. (2013). Transposition of native chromatin for fast and sensitive epigenomic profiling of open chromatin, DNA-binding proteins and nucleosome position. Nat. Methods 10, 1213–1218. doi:10.1038/nmeth.2688
Buenrostro, J. D., Wu, B., Litzenburger, U. M., Ruff, D., Gonzales, M. L., Snyder, M. P., et al. (2015). Single-cell chromatin accessibility reveals principles of regulatory variation. Nature 523, 486–490. doi:10.1038/nature14590
Cao, J., Cusanovich, D. A., Ramani, V., Aghamirzaie, D., Pliner, H. A., Hill, A. J., et al. (2018). Joint profiling of chromatin accessibility and gene expression in thousands of single cells. Science 361, 1380–1385. doi:10.1126/science.aau0730
Cao, J., Zhou, W., Steemers, F., Trapnell, C., and Shendure, J. (2020). Sci-fate characterizes the dynamics of gene expression in single cells. Nat. Biotechnol. 38, 980–988. doi:10.1038/s41587-020-0480-9
Chappell, L., Russell, A. J. C., and Voet, T. (2018). Single-cell (Multi)omics technologies. Annu. Rev. Genomics Hum. Genet. 19, 15–41. doi:10.1146/annurev-genom-091416-035324
Chen, A. F., Parks, B., Kathiria, A. S., Ober-Reynolds, B., Goronzy, J. J., and Greenleaf, W. J. (2022a). NEAT-Seq: Simultaneous profiling of intra-nuclear proteins, chromatin accessibility and gene expression in single cells. Nat. Methods 19, 547–553. doi:10.1038/s41592-022-01461-y
Chen, S., Lake, B. B., and Zhang, K. (2019). High-throughput sequencing of the transcriptome and chromatin accessibility in the same cell. Nat. Biotechnol. 37, 1452–1457. doi:10.1038/s41587-019-0290-0
Chen, W., Guillaume-Gentil, O., Rainer, P. Y., Gabelein, C. G., Saelens, W., Gardeux, V., et al. (2022b). Live-seq enables temporal transcriptomic recording of single cells. Nature 608, 733–740. doi:10.1038/s41586-022-05046-9
Cusanovich, D. A., Daza, R., Adey, A., Pliner, H. A., Christiansen, L., Gunderson, K. L., et al. (2015). Multiplex single cell profiling of chromatin accessibility by combinatorial cellular indexing. Science 348, 910–914. doi:10.1126/science.aab1601
Dey, S. S., Kester, L., Spanjaard, B., Bienko, M., and van Oudenaarden, A. (2015). Integrated genome and transcriptome sequencing of the same cell. Nat. Biotechnol. 33, 285–289. doi:10.1038/nbt.3129
Efremova, M., and Teichmann, S. A. (2020). Computational methods for single-cell omics across modalities. Nat. Methods 17, 14–17. doi:10.1038/s41592-019-0692-4
Gopalan, S., Wang, Y., Harper, N. W., Garber, M., and Fazzio, T. G. (2021). Simultaneous profiling of multiple chromatin proteins in the same cells. Mol. Cell 81, 4736–4746.e5. doi:10.1016/j.molcel.2021.09.019
Greenberg, M. V. C., and Bourc'his, D. (2019). The diverse roles of DNA methylation in mammalian development and disease. Nat. Rev. Mol. Cell Biol. 20, 590–607. doi:10.1038/s41580-019-0159-6
Group, P. T. C., Calabrese, C., Davidson, N. R., Demircioglu, D., Fonseca, N. A., He, Y., et al. (2020). Genomic basis for RNA alterations in cancer. Nature 578, 129–136. doi:10.1038/s41586-020-1970-0
Hashimshony, T., Wagner, F., Sher, N., and Yanai, I. (2012). CEL-seq: Single-cell RNA-seq by multiplexed linear amplification. Cell Rep. 2, 666–673. doi:10.1016/j.celrep.2012.08.003
Hasin-Brumshtein, Y., Lancet, D., and Olender, T. (2009). Human olfaction: From genomic variation to phenotypic diversity. Trends Genet. 25, 178–184. doi:10.1016/j.tig.2009.02.002
Hou, Y., Guo, H., Cao, C., Li, X., Hu, B., Zhu, P., et al. (2016). Single-cell triple omics sequencing reveals genetic, epigenetic, and transcriptomic heterogeneity in hepatocellular carcinomas. Cell Res. 26, 304–319. doi:10.1038/cr.2016.23
Hu, Y., Huang, K., An, Q., Du, G., Hu, G., Xue, J., et al. (2016). Simultaneous profiling of transcriptome and DNA methylome from a single cell. Genome Biol. 17, 88. doi:10.1186/s13059-016-0950-z
Janssens, D. H., Otto, D. J., Meers, M. P., Setty, M., Ahmad, K., and Henikoff, S. (2022). CUT&Tag2for1: A modified method for simultaneous profiling of the accessible and silenced regulome in single cells. Genome Biol. 23, 81. doi:10.1186/s13059-022-02642-w
Kaya-Okur, H. S., Wu, S. J., Codomo, C. A., Pledger, E. S., Bryson, T. D., Henikoff, J. G., et al. (2019). CUT&Tag for efficient epigenomic profiling of small samples and single cells. Nat. Commun. 10, 1930. doi:10.1038/s41467-019-09982-5
Lake, B. B., Chen, S., Sos, B. C., Fan, J., Kaeser, G. E., Yung, Y. C., et al. (2018). Integrative single-cell analysis of transcriptional and epigenetic states in the human adult brain. Nat. Biotechnol. 36, 70–80. doi:10.1038/nbt.4038
Lareau, C. A., Duarte, F. M., Chew, J. G., Kartha, V. K., Burkett, Z. D., Kohlway, A. S., et al. (2019). Droplet-based combinatorial indexing for massive-scale single-cell chromatin accessibility. Nat. Biotechnol. 37, 916–924. doi:10.1038/s41587-019-0147-6
Lee, D. S., Luo, C., Zhou, J., Chandran, S., Rivkin, A., Bartlett, A., et al. (2019). Simultaneous profiling of 3D genome structure and DNA methylation in single human cells. Nat. Methods 16, 999–1006. doi:10.1038/s41592-019-0547-z
Li, G., Liu, Y., Zhang, Y., Kubo, N., Yu, M., Fang, R., et al. (2019). Joint profiling of DNA methylation and chromatin architecture in single cells. Nat. Methods 16, 991–993. doi:10.1038/s41592-019-0502-z
Lieberman-Aiden, E., van Berkum, N. L., Williams, L., Imakaev, M., Ragoczy, T., Telling, A., et al. (2009). Comprehensive mapping of long-range interactions reveals folding principles of the human genome. Science 326, 289–293. doi:10.1126/science.1181369
Liu, L., Liu, C., Quintero, A., Wu, L., Yuan, Y., Wang, M., et al. (2019). Deconvolution of single-cell multi-omics layers reveals regulatory heterogeneity. Nat. Commun. 10, 470. doi:10.1038/s41467-018-08205-7
Luo, C., Liu, H., Xie, F., Armand, E. J., Siletti, K., Bakken, T. E., et al. (2022). Single nucleus multi-omics identifies human cortical cell regulatory genome diversity. Cell Genom 2, 100107. doi:10.1016/j.xgen.2022.100107
Ma, S., Zhang, B., LaFave, L. M., Earl, A. S., Chiang, Z., Hu, Y., et al. (2020). Chromatin potential identified by shared single-cell profiling of RNA and chromatin. Cell 183, 1103–1116. doi:10.1016/j.cell.2020.09.056
Macaulay, I. C., Haerty, W., Kumar, P., Li, Y. I., Hu, T. X., Teng, M. J., et al. (2015). G&T-seq: Parallel sequencing of single-cell genomes and transcriptomes. Nat. Methods 12, 519–522. doi:10.1038/nmeth.3370
Mateo, L. J., Murphy, S. E., Hafner, A., Cinquini, I. S., Walker, C. A., and Boettiger, A. N. (2019). Visualizing DNA folding and RNA in embryos at single-cell resolution. Nature 568, 49–54. doi:10.1038/s41586-019-1035-4
Meers, M. P., Llagas, G., Janssens, D. H., Codomo, C. A., and Henikoff, S. (2022). Multifactorial profiling of epigenetic landscapes at single-cell resolution using MulTI-Tag. Nat. Biotechnol. 2022. doi:10.1038/s41587-022-01522-9
Mezger, A., Klemm, S., Mann, I., Brower, K., Mir, A., Bostick, M., et al. (2018). High-throughput chromatin accessibility profiling at single-cell resolution. Nat. Commun. 9, 3647. doi:10.1038/s41467-018-05887-x
Mimitou, E. P., Lareau, C. A., Chen, K. Y., Zorzetto-Fernandes, A. L., Hao, Y., Takeshima, Y., et al. (2021). Scalable, multimodal profiling of chromatin accessibility, gene expression and protein levels in single cells. Nat. Biotechnol. 39, 1246–1258. doi:10.1038/s41587-021-00927-2
Nagano, T., Lubling, Y., Stevens, T. J., Schoenfelder, S., Yaffe, E., Dean, W., et al. (2013). Single-cell Hi-C reveals cell-to-cell variability in chromosome structure. Nature 502, 59–64. doi:10.1038/nature12593
Papalexi, E., and Satija, R. (2018). Single-cell RNA sequencing to explore immune cell heterogeneity. Nat. Rev. Immunol. 18, 35–45. doi:10.1038/nri.2017.76
Peterson, V. M., Zhang, K. X., Kumar, N., Wong, J., Li, L., Wilson, D. C., et al. (2017). Multiplexed quantification of proteins and transcripts in single cells. Nat. Biotechnol. 35, 936–939. doi:10.1038/nbt.3973
Qiu, Q., Hu, P., Qiu, X., Govek, K. W., Camara, P. G., and Wu, H. (2020). Massively parallel and time-resolved RNA sequencing in single cells with scNT-seq. Nat. Methods 17, 991–1001. doi:10.1038/s41592-020-0935-4
Ramani, V., Deng, X., Qiu, R., Gunderson, K. L., Steemers, F. J., Disteche, C. M., et al. (2017). Massively multiplex single-cell Hi-C. Nat. Methods 14, 263–266. doi:10.1038/nmeth.4155
Rang, F. J., de Luca, K. L., de Vries, S. S., Valdes-Quezada, C., Boele, E., Nguyen, P. D., et al. (2022). Single-cell profiling of transcriptome and histone modifications with EpiDamID. Mol. Cell 82, 1956–1970.e14. doi:10.1016/j.molcel.2022.03.009
Rotem, A., Ram, O., Shoresh, N., Sperling, R. A., Goren, A., Weitz, D. A., et al. (2015). Single-cell ChIP-seq reveals cell subpopulations defined by chromatin state. Nat. Biotechnol. 33, 1165–1172. doi:10.1038/nbt.3383
Schlesinger, F., Smith, A. D., Gingeras, T. R., Hannon, G. J., and Hodges, E. (2013). De novo DNA demethylation and noncoding transcription define active intergenic regulatory elements. Genome Res. 23, 1601–1614. doi:10.1101/gr.157271.113
Smallwood, S. A., Lee, H. J., Angermueller, C., Krueger, F., Saadeh, H., Peat, J., et al. (2014). Single-cell genome-wide bisulfite sequencing for assessing epigenetic heterogeneity. Nat. Methods 11, 817–820. doi:10.1038/nmeth.3035
Smith, Z. D., and Meissner, A. (2013). DNA methylation: Roles in mammalian development. Nat. Rev. Genet. 14, 204–220. doi:10.1038/nrg3354
Stevens, T. J., Lando, D., Basu, S., Atkinson, L. P., Cao, Y., Lee, S. F., et al. (2017). 3D structures of individual mammalian genomes studied by single-cell Hi-C. Nature 544, 59–64. doi:10.1038/nature21429
Stoeckius, M., Hafemeister, C., Stephenson, W., Houck-Loomis, B., Chattopadhyay, P. K., Swerdlow, H., et al. (2017). Simultaneous epitope and transcriptome measurement in single cells. Nat. Methods 14, 865–868. doi:10.1038/nmeth.4380
Stuart, T., Hao, S., Zhang, B., Mekerishvili, L., Landau, D. A., Maniatis, S., et al. (2022). Nanobody-tethered transposition enables multifactorial chromatin profiling at single-cell resolution. Nat. Biotechnol. 2022. doi:10.1038/s41587-022-01588-5
Swanson, E., Lord, C., Reading, J., Heubeck, A. T., Genge, P. C., Thomson, Z., et al. (2021). Simultaneous trimodal single-cell measurement of transcripts, epitopes, and chromatin accessibility using TEA-seq. Elife 10, e63632. doi:10.7554/eLife.63632
Tang, F., Barbacioru, C., Wang, Y., Nordman, E., Lee, C., Xu, N., et al. (2009). mRNA-Seq whole-transcriptome analysis of a single cell. Nat. Methods 6, 377–382. doi:10.1038/nmeth.1315
Tedesco, M., Giannese, F., Lazarevic, D., Giansanti, V., Rosano, D., Monzani, S., et al. (2022). Chromatin Velocity reveals epigenetic dynamics by single-cell profiling of heterochromatin and euchromatin. Nat. Biotechnol. 40, 235–244. doi:10.1038/s41587-021-01031-1
Vickovic, S., Lötstedt, B., Klughammer, J., Mages, S., Segerstolpe, Å., Rozenblatt-Rosen, O., et al. (2022). SM-Omics is an automated platform for high-throughput spatial multi-omics. Nat. Commun. 13 (1), 795
Wagner, D. E., and Klein, A. M. (2020). Lineage tracing meets single-cell omics: Opportunities and challenges. Nat. Rev. Genet. 21, 410–427. doi:10.1038/s41576-020-0223-2
Wu, S. J., Furlan, S. N., Mihalas, A. B., Kaya-Okur, H. S., Feroze, A. H., Emerson, S. N., et al. (2021). Single-cell CUT&Tag analysis of chromatin modifications in differentiation and tumor progression. Nat. Biotechnol. 39, 819–824. doi:10.1038/s41587-021-00865-z
Xiong, H., Luo, Y., Wang, Q., Yu, X., and He, A. (2021). Single-cell joint detection of chromatin occupancy and transcriptome enables higher-dimensional epigenomic reconstructions. Nat. Methods 18, 652–660. doi:10.1038/s41592-021-01129-z
Xu, W., Yang, W., Zhang, Y., Chen, Y., Hong, N., Zhang, Q., et al. (2022). ISSAAC-seq enables sensitive and flexible multimodal profiling of chromatin accessibility and gene expression in single cells. Nat. Methods 19, 1243–1249. doi:10.1038/s41592-022-01601-4
Yeung, J., Florescu, M., Zeller, P., de Barbanson, B. A., Wellenstein, M. D., and van Oudenaarden, A. (2023). scChIX-seq infers dynamic relationships between histone modifications in single cells. Nat. Biotechnol. 2023. doi:10.1038/s41587-022-01560-3
Zhang, B., Srivastava, A., Mimitou, E., Stuart, T., Raimondi, I., Hao, Y., et al. (2022). Characterizing cellular heterogeneity in chromatin state with scCUT&Tag-pro. Nat. Biotechnol. 40, 1220–1230. doi:10.1038/s41587-022-01250-0
Zhang, D., Deng, Y., Kukanja, P., Agirre, E., Bartosovic, M., Dong, M., et al. (2023). Spatial epigenome-transcriptome co-profiling of mammalian tissues. Nature 616, 113–122. doi:10.1038/s41586-023-05795-1
Zhang, F., Gu, W., Hurles, M. E., and Lupski, J. R. (2009). Copy number variation in human health, disease, and evolution. Annu. Rev. Genomics Hum. Genet. 10, 451–481. doi:10.1146/annurev.genom.9.081307.164217
Zhu, C., Preissl, S., and Ren, B. (2020). Single-cell multimodal omics: The power of many. Nat. Methods 17, 11–14. doi:10.1038/s41592-019-0691-5
Zhu, C., Yu, M., Huang, H., Juric, I., Abnousi, A., Hu, R., et al. (2019). An ultra high-throughput method for single-cell joint analysis of open chromatin and transcriptome. Nat. Struct. Mol. Biol. 26, 1063–1070. doi:10.1038/s41594-019-0323-x
Zhu, C., Zhang, Y., Li, Y. E., Lucero, J., Behrens, M. M., and Ren, B. (2021). Joint profiling of histone modifications and transcriptome in single cells from mouse brain. Nat. Methods 18, 283–292. doi:10.1038/s41592-021-01060-3
No hay comentarios:
Publicar un comentario