La IA rastrea misteriosos cánceres metastásicos hasta su origen
AI traces mysterious metastatic cancers to their source
Un algoritmo examina imágenes de células metastásicas para identificar la ubicación del tumor primario.
Nature presenta una Inteligencia Artificial entrenada pra rastrear cánceres metastásicos hasta su origen. Un reciente estudio publicado en Nature por investigadores de la Universidad Médica de Tianjin ha dado un paso gigante hacia la mejora del diagnóstico de cánceres metastásicos. Este estudio desarrolló una herramienta de inteligencia artificial que no solo iguala, sino que supera la capacidad de los patólogos para identificar los orígenes de células cancerosas metastásicas. Este modelo de IA, entrenado con cerca de 30,000 imágenes de células cancerosas, muestra una precisión del 83% en predecir el origen del tumor y un 99% de posibilidad de que el origen esté en sus tres principales predicciones. Esta herramienta es un avance significativo porque puede reducir la necesidad de pruebas adicionales, a menudo intrusivas, que los pacientes deben soportar.
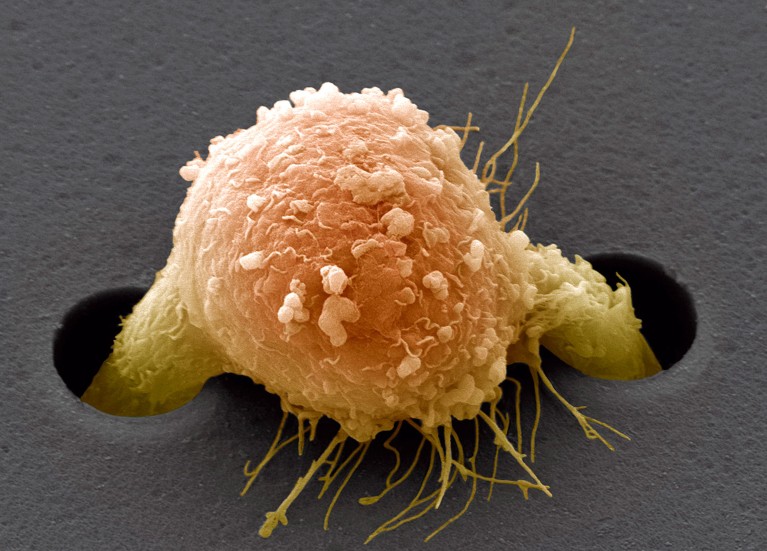
A breast cancer cell (artificially coloured) climbs through a supportive film in a laboratory experiment.Credit: Steve Gschmeissner/SPL
Some stealthy cancers remain undetected until they have spread from their source to distant organs. Now scientists have developed an artificial intelligence (AI) tool that outperforms pathologists at identifying the origins of metastatic cancer cells that circulate in the body1. The proof-of-concept model could help doctors to improve the diagnosis and treatment of late-stage cancer, and extend people’s lives.
“That’s a pretty significant finding — that it can be used as an assistive tool,” says Faisal Mahmood, who studies AI applications in health care at Harvard Medical School in Boston, Massachusetts.
Elusive origins
To treat metastatic cancers, doctors need to know where they came from. The origin of up to 5% of all tumours cannot be identified, and the prognosis for people whose primary cancer remains unknown is poor.
One method used to diagnose tricky metastatic cancers relies on tumour cells found in fluid extracted from the body. Clinicians examine images of the cells to work out which type of cancer cell they resemble. For example, breast cancer cells that migrate to the lungs still look like breast cancer cells.
Every year, of the 300,000 people with cancer who are newly treated at the hospital affiliated with Tianjin Medical University (TMU) in China, some 4,000 are diagnosed using such images, but around 300 people remain undiagnosed, says Tian Fei, a colorectal cancer surgeon at TMU.
Tian, Li Xiangchun, a bioinformatics researcher who studies deep learning at TMU, and their colleagues wanted to develop a deep-learning algorithm to analyse these images and predict the origin of the cancers. Their results were published in Nature Medicine on 16 April.
Tumour training
The researchers trained their AI model on some 30,000 images of cells found in abdominal or lung fluid from 21,000 people whose tumour of origin was known. They then tested their model on 27,000 images and found there was an 83% chance that it would accurately predict the source of the tumour. And there was a 99% chance that the source of the tumour was included in the model’s top three predictions.
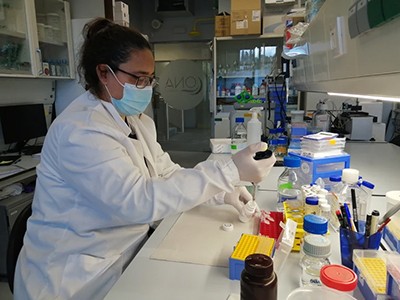
ONA Therapeutics: A fat-blocking drug could help to fight metastatic cancer
Having a top-three list is useful because it can help clinicians to reduce the number of extra — often intrusive — tests needed to identify a tumour’s origins, says Mahmood. The predictions were restricted to 12 common sources of cancer, including the lungs, ovaries, breasts and stomach. Some other forms of cancer, including those originating in the prostate and kidneys, could not be identified, because they don’t typically spread to fluid deposits in the abdomen and lungs, says Li.
When tested on some 500 images, the model was better than human pathologists at predicting a tumour’s origin. This improvement was statistically significant.
The researchers also retrospectively assessed a subset of 391 study participants some four years after they had had cancer treatment. They found that those who had received treatment for the type of cancer that the model predicted were more likely to have survived, and lived longer, than participants for whom the prediction did not match. “This is a pretty convincing argument” for using the AI model in a clinical setting, says Mahmood.
Mahmood has previously used AI to predict the origin of cancers from tissue samples2, and other teams have used genomic data. Combining the three data sources — cells, tissue and genomics — could further improve outcomes for people with metastatic cancers of unknown origins, he says.
Nature 628, 699-700 (2024)
doi: https://doi.org/10.1038/d41586-024-01110-8
References
Tian, F. et al. Nature Med. https://doi.org/10.1038/s41591-024-02915-w (2024).
Lu, M. Y. et al. Nature 594, 106–110 (2021).
No hay comentarios:
Publicar un comentario